We have witnessed the past decades tremendous progress made in machine learning including deep learning recently. Machine learning is now used in many tasks that affect our daily life and is currently making strives regarding our health. Yet, little has been done to interface machine learning with biological systems at the molecular level, this is noteworthy considering that deep learning methods are inspired by the neural network of our brain.
Using artificial neural network for diagnosis has been undertaken for many diseases, however, one needs biomarkers concentrations to both train the network and use it for diagnosis. Taking raw clinical samples as network input and producing an output that can directly be measured requires to construct the network in a biological system. A research team led by Prof. Faulon (SYNBIOCHEM center) has just taken a first step in this direction by engineering a simple neural network in an E. coli cell-free extract1. The network classifies samples having different metabolic compositions. The network is trained in silico and actuated in a cell extract through the modulation of enzyme concentrations. In a second study2, Faulon’s team partnered with J. Bonnet’s group at INSERM (France) and performed validation tests on human urine for metabolites detection. Among the selected metabolites, hippurate was chosen because it can be used to monitor a recent probiotic treatment of phenylketonuria (a neurotoxic disease). Indeed, Isabella et al. (Nat. Biotechnol. 2018) have recently shown that adding a lyase to a probiotic (E. coli Nissle 1917) produces hippurate while treating the disease. Thus, engineering a low-cost device based on E. coli cell extract enables one to monitor treatment efficacy avoiding more expensive and time-consuming mass spectrometry measurements.
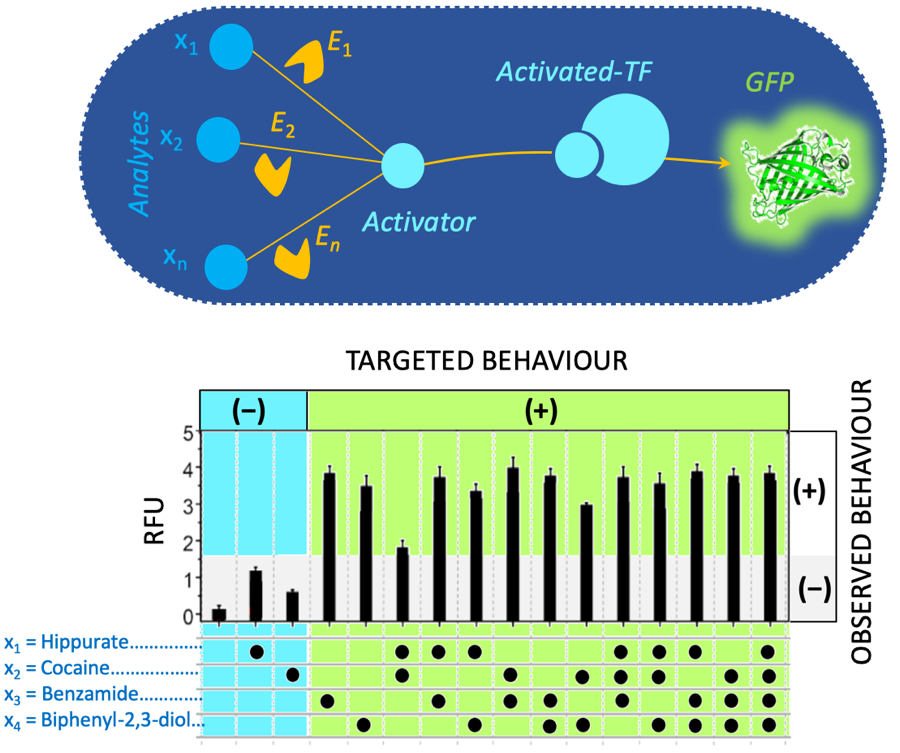
Engineering a simple neural network in a E. coli cell extract. The network is a one-layer perceptron. It can be used for classification (output is negative – or positive +). The network is composed of enzymes (yellow) that transform analytes into an activator (light blue). The activator binds a transcription factor (TF) to express a reporter gene (GFP). The graph at the bottom plots the fluorescence measurements (RFU: Relative Fluorescence Unit) of a 4-input perceptron (hippurate, cocaine, benzamide and biphenyl-2,3-diol). Among the 16 possible configurations (presence or absence of one or several inputs) the targeted behavior of the first 3 configurations is negative while others are expected to be positive. The observed behavior (black vertical bars) matches well the targeted behavior.
References
- Pandi, A. et al. Metabolic Perceptrons for Neural Computing in Biological Systems. Nat. Commun. 10, 3880 (2019). doi: 10.1038/s41467-019-11889-0
- Voyvodic, P. L. et al. Plug-and-play metabolic transducers expand the chemical detection space of cell-free biosensors. Nat. Commun. 10, 1697 (2019). doi: 10.1038/s41467-019-09722-9